One of the main things we believe in at Tablecloth is getting beyond self-reported metrics, searching instead for high-quality data where it already exists in the enterprise.
The importance of high-quality ESG data for investors and stakeholders
With such high stakes in ESG (our people and planet depend upon getting the right answers ASAP), I would say high-quality data is not just a "nice-to-have"—but a necessity. High-quality ESG data is critical for investors and stakeholders who rely on this information to make informed decisions on investments, engagement, and advocacy.
Low-quality data, on the other hand, has errors, duplicates, and inconsistencies. Low-quality data might also be irrelevant and/or out-of-date; i.e. you’re looking at the wrong data. For high-quality decision-making, let’s stick to high-quality data.

Best practices for ensuring high-quality data
When we are looking at our data, we evaluate it according these five characteristics:
Accuracy: The degree to which data reflects the real world.
Completeness: The extent to which all required data elements are present.
Consistency: The degree to which data conforms to defined rules or relationships.
Validity: The degree to which data meets specific criteria or standards.
Timeliness: The degree to which data is up-to-date and relevant to the current context.
Below are some helpful guidelines for achieving all of the above. Let’s have a look.
Data collection
Be deliberate about your collection methods. Ensure that the data collection methods are consistent, reliable, and unbiased. Data collection methods should follow best practices, be well-documented, and be periodically reviewed for potential improvements. If needed, expand the scope of data collection to cover any gaps and account for any potential missing data.
Improves: Accuracy. Completeness. Validity. Timeliness.
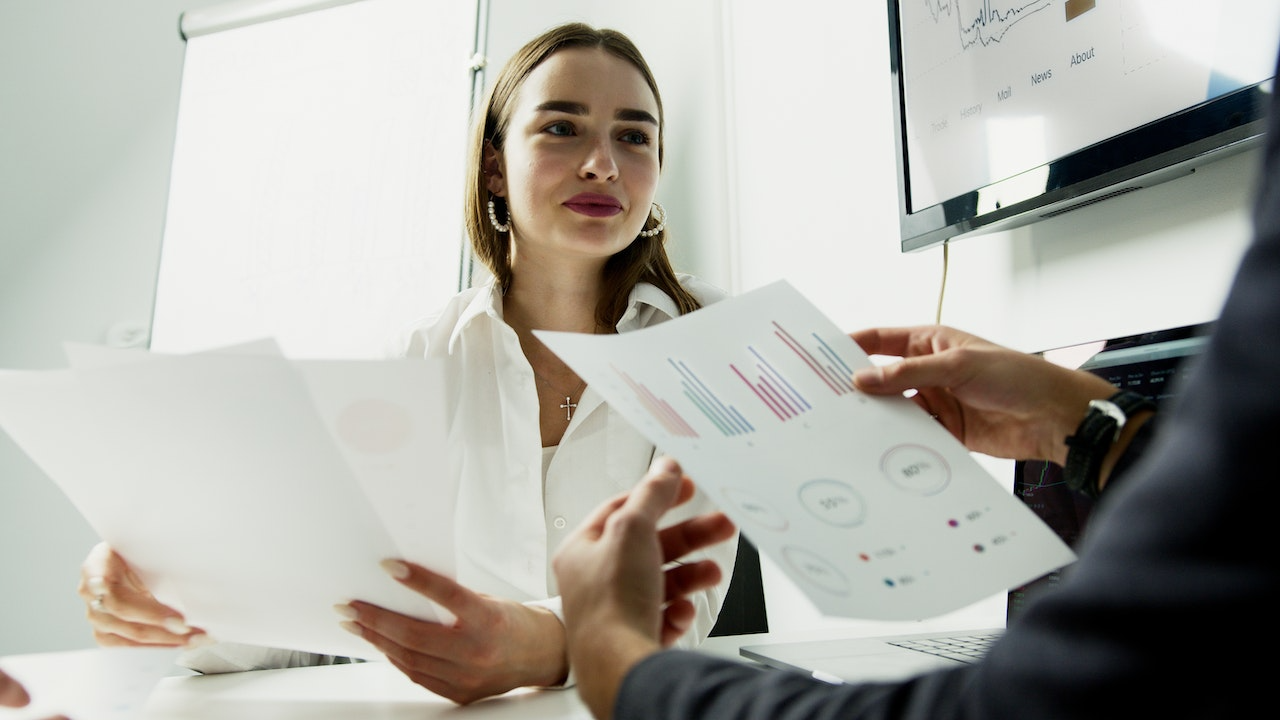
Data validation (at entry and at rest)
Implement data entry validation checks and constraints to minimize errors, such as duplicate entries or incorrect formats. Use techniques like cross-validation or limit checks to ensure the data entered is within the acceptable range. Implement data validation rules to ensure that all required fields are populated during data entry. Check results at rest to verify front-end validations are working.
Improves: Accuracy. Consistency. Validity.
Data profiling
Perform data profiling to assess the completeness of your data. This involves examining the distribution of values, the presence of null or empty values, and any patterns of missing data.
Improves: Accuracy. Completeness. Consistency. Validity.
Data cleansing
Regularly clean and preprocess the data to remove inaccuracies, inconsistencies, and duplicates. Use tools and techniques like data profiling, standardization, and transformation to improve data quality.
Improves: Accuracy. Consistency. Validity.
Data imputation
Use data imputation techniques to fill in missing values when appropriate. Techniques include mean imputation, median imputation, regression imputation, and more advanced methods like k-nearest neighbors and machine learning algorithms.
Improves: Completeness.

Data source verification
Verify the reliability of data sources and ensure they are reputable, accurate, and up-to-date. Cross-reference multiple sources when possible to ensure consistency and completeness.
Improves: Accuracy. Completeness. Consistency. Validity. Timeliness.
Data audits
Periodically audit the data to identify anomalies, inconsistencies, or inaccuracies. Use statistical techniques like outlier detection, data profiling, or data lineage analysis to identify and correct errors.
Improves: Accuracy. Completeness. Validity. Timeliness.
Data governance
Implement a robust data governance framework to ensure data accuracy, including clearly defined roles and responsibilities, data quality policies, and data lineage documentation.
Improves: Accuracy. Completeness. Consistency. Validity. Timeliness.
Training and communication
Train team members on data quality best practices, and encourage open communication about potential data issues. Encourage a culture of data quality awareness and continuous improvement.
Improves: Accuracy. Consistency. Validity. Timeliness.
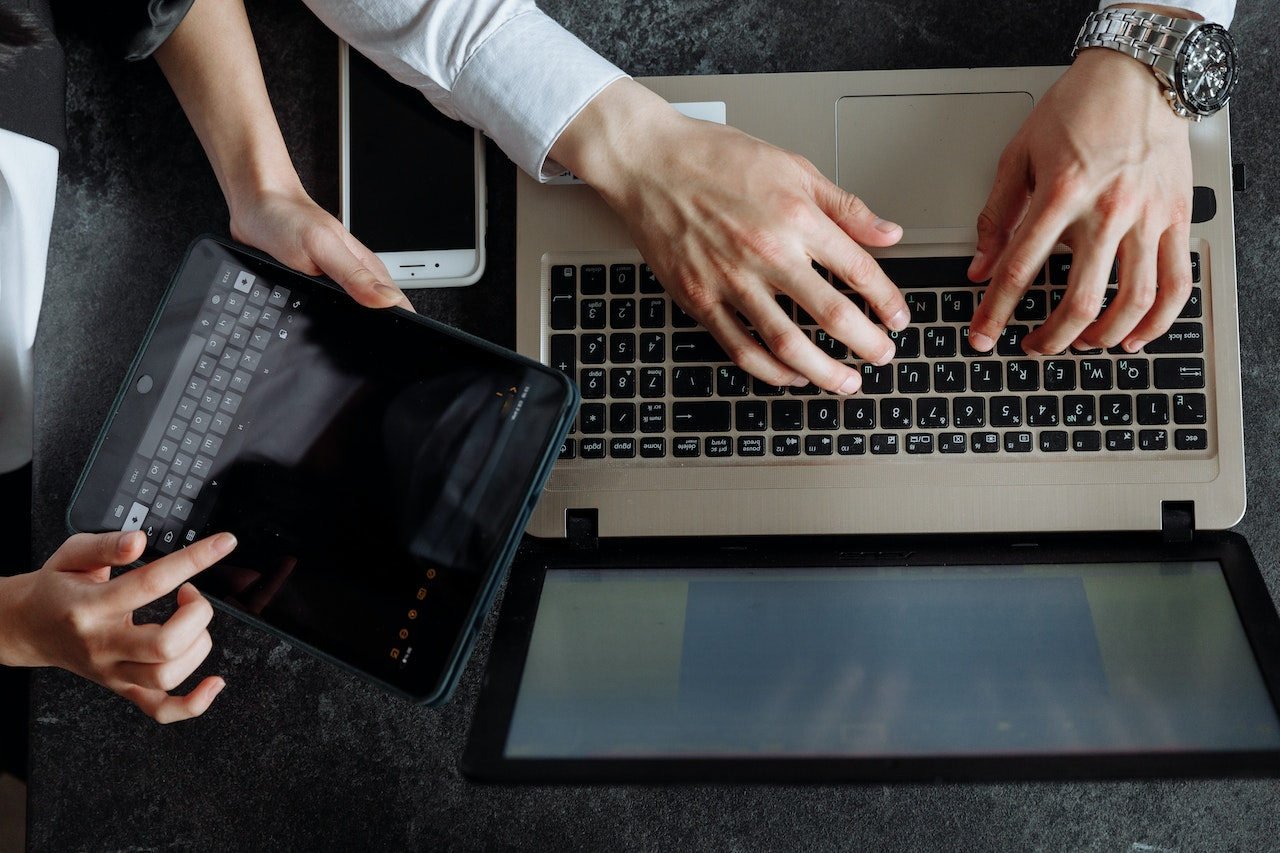
Metadata management
Maintain accurate and up-to-date metadata to provide context for data interpretation and analysis. Metadata should include information on data origin, data lineage, data definitions, and data transformations.
Improves: Accuracy.
External validation
When possible, validate your data against external benchmarks, industry standards, or third-party sources. This can help identify inaccuracies and provide additional confidence in the quality of your data.
Improves: Accuracy.
Feedback loops
Implement feedback loops with data providers and users to continually improve data completeness. Encourage stakeholders to report issues or suggest improvements, and act on their feedback to enhance the quality of your data.
Improves: Completeness.
Data standardization
Establish data standards and guidelines for data entry, including consistent formats, units of measurement, and naming conventions. Consistently adhering to these standards can help prevent discrepancies and improve data quality.
Improves: Consistency.
Data transformation
Apply consistent data transformation processes when converting or modifying data, such as changing data types, aggregating data, or applying calculations. Document these processes to ensure that they are consistently followed.
Improves: Consistency.

Data update frequency
Establish a clear schedule for updating your data, based on the needs of your analysis and the rate at which the data changes. Regularly review and adjust the update frequency as necessary to maintain data relevance.
Improves: Timeliness.
Data processing efficiency
Optimize your data processing workflows to minimize the time it takes to process, clean, and integrate new data. Use efficient data storage and processing technologies, and automate data processing tasks when possible.
Improves: Timeliness
Data monitoring and alerting
Implement monitoring and alerting systems to notify you of data updates, delays, or issues that may impact data timeliness. Use these alerts to take corrective actions and maintain data freshness.
Improves: Timeliness